How Automation Is Speeding Up, speed and efficiency are crucial to staying competitive. Consumers and businesses alike demand fast and seamless services, and the credit industry is no exception. The traditional credit approval process, often slow and cumbersome, has become a target for disruption. As financial technology (fintech) evolves, automation has emerged as a game-changer, speeding up credit approval, reducing manual errors, and enhancing the overall customer experience. Automation is reshaping how credit is assessed, granted, and processed, allowing financial institutions to provide quicker, more efficient services while maintaining a high level of accuracy and security.
This article explores the role of automation in accelerating the credit approval process, highlighting key benefits, technologies, and challenges that come with automating this crucial aspect of the financial sector.
1. The Traditional Credit Approval Process: Slow and Complex
Before the rise of automation, the credit approval process was often slow and filled with manual steps. Here’s a brief overview of the traditional process:
- Application Submission: Borrowers would typically submit a paper-based or online application, often requiring personal information, financial records, and additional documentation.
- Manual Review: A credit analyst would review the application, manually checking the borrower’s credit history, financial status, and supporting documents.
- Verification: The approval process also involved verifying the borrower’s information with third-party sources, such as credit bureaus, banks, or other financial institutions.
- Decision Making: The credit analyst would then make a final decision, which might involve discussions or meetings with supervisors, leading to a delay in processing.
- Notification: Finally, the borrower would receive an approval or denial, often days or even weeks after the application was first submitted.
In this traditional system, several factors contributed to delays, including human error, back-and-forth communication, and the heavy reliance on paper documentation. As demand for faster, more responsive services grew, it became clear that automation could provide a solution to these inefficiencies.
2. The Rise of Automation in Credit Approvals
Automation in the credit approval process involves using technology to streamline tasks, minimize human intervention, and speed up decision-making. Today’s automated systems use artificial intelligence (AI), machine learning (ML), data analytics, and workflow automation tools to handle many aspects of credit evaluation more quickly and accurately than ever before.
2.1 Key Technologies Behind Automation
To understand how automation is transforming credit approvals, it is essential to look at the technologies driving this change:
-
Artificial Intelligence (AI): AI is at the core of many credit automation systems. AI models can process large amounts of data, identify patterns, and make decisions in a fraction of the time it would take a human. AI systems can analyze credit scores, payment histories, income data, and other financial indicators to provide a quick risk assessment.
-
Machine Learning (ML): ML, a subset of AI, allows credit approval systems to “learn” from historical data and continuously improve their decision-making capabilities. Over time, ML algorithms can predict creditworthiness with greater accuracy by factoring in more variables and adapting to changes in market conditions.
-
Robotic Process Automation (RPA): RPA automates repetitive, rule-based tasks. For example, it can automate data entry, verify documents, or pull credit reports from bureaus, reducing the time spent on manual, low-value tasks.
-
Application Programming Interfaces (APIs): APIs allow different systems to communicate with each other in real time. In the context of credit approval, APIs can automatically fetch financial data, verify identities, or check credit histories with third-party services, speeding up the decision-making process.
-
Big Data Analytics: Big data enables financial institutions to analyze vast amounts of data from multiple sources. By leveraging advanced analytics, banks and lenders can gain deeper insights into a borrower’s financial profile, allowing for faster and more informed decisions.
These technologies, working in tandem, enable automation to handle complex tasks that were once time-consuming and prone to error.
3. Benefits of Automation in Credit Approval
The adoption of automation in credit approval offers numerous advantages for both consumers and financial institutions.
3.1 Faster Processing Time
The most immediate benefit of automation is the significant reduction in processing time. Tasks that once took days or even weeks, such as manual data entry, document verification, and credit analysis, can now be completed in a matter of minutes. For example, AI algorithms can instantly analyze a borrower’s creditworthiness by accessing multiple data sources and generating a credit decision within seconds.
This speed is especially crucial in the consumer credit sector, where customers expect instant approvals for credit cards, personal loans, or mortgages. Automation ensures that lenders can meet these expectations without compromising on the quality of decision-making.
3.2 Reduced Human Error
Manual processes are inherently prone to human error, from data entry mistakes to incorrect analysis or misinterpretation of information. Automation minimizes the risk of such errors by standardizing tasks and ensuring consistency in decision-making.
For instance, an AI model used to assess creditworthiness can make decisions based on pre-defined criteria, reducing bias and subjectivity in the evaluation process. Automated systems also help reduce the risk of fraudulent activities by detecting inconsistencies in data and flagging suspicious activity immediately.
3.3 Cost Savings for Financial Institutions
By reducing the need for manual labor and streamlining the credit approval process, automation helps financial institutions cut operational costs. Staff no longer have to spend time on repetitive tasks like checking documents, inputting data, or making decisions manually. Instead, automated systems can handle these tasks with higher efficiency, allowing human workers to focus on higher-value activities, such as client relationships and strategic decision-making.
Additionally, the quicker approval process means that lenders can process a higher volume of applications, increasing their revenue potential without increasing overhead costs.
3.4 Enhanced Customer Experience
With automated systems, consumers can experience faster credit approvals, more personalized offers, and fewer administrative hurdles. For example, AI can quickly analyze a borrower’s financial behavior and recommend credit products that best match their needs and credit profile. This creates a more streamlined, user-friendly experience, which can improve customer satisfaction and loyalty.
Automated systems can also be integrated into mobile apps and online platforms, allowing consumers to apply for credit and track the status of their application in real-time, 24/7.
3.5 Better Risk Management
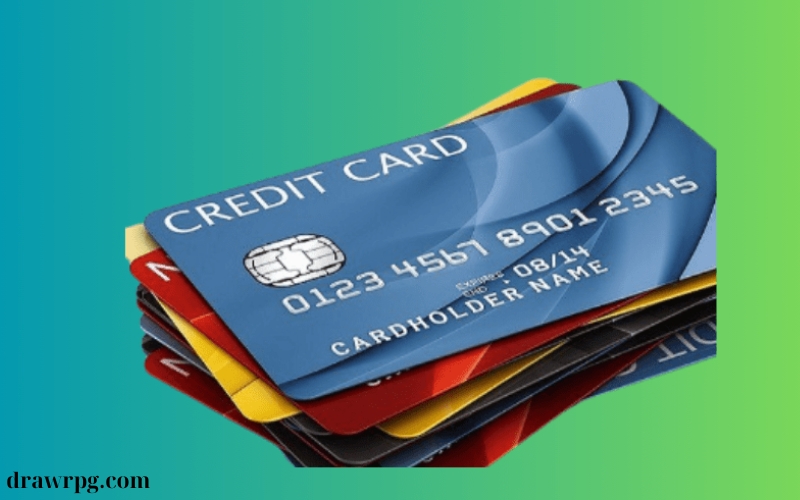
How Automation Is Speeding Up more informed decisions about credit risk. By using advanced algorithms and machine learning, automated systems can analyze vast amounts of data to predict a borrower’s likelihood of default. These systems can take into account a wider range of variables than traditional methods, including alternative data sources like social media activity, utility payments, and even educational background.
In this way, automation allows lenders to assess credit risk more accurately and make better lending decisions, ultimately reducing default rates and enhancing the stability of financial institutions.
4. How Automation Works in the Credit Approval Process
Let’s take a closer look at the step-by-step process of how automation is applied to credit approvals.
4.1 Application Submission and Data Collection
When a consumer applies for credit, the application can be submitted online or through a mobile app. Automation tools immediately collect and store the data provided, such as personal information, financial details, and supporting documents.
Using APIs, the system can then cross-check this information with third-party databases, such as credit bureaus or public records, to verify the data and assess the borrower’s creditworthiness.
4.2 Credit Scoring and Risk Assessment
Once the data is collected, the automated system uses AI and machine learning models to analyze the borrower’s credit history, financial behavior, and other relevant factors. Traditional credit scoring systems, such as FICO, can be enhanced with alternative data sources, allowing lenders to assess individuals who may have little or no formal credit history.
The system can also calculate the borrower’s credit risk in real-time, using predictive models to determine the likelihood of repayment based on past behavior, economic trends, and other factors.
4.3 Decision Making
How Automation Is Speeding Up, the automated system makes a decision on whether to approve or deny the loan. In many cases, this decision can be made instantly, without the need for human intervention. However, more complex cases may still require manual review by a credit analyst.
If the loan is approved, the system can automatically generate a loan agreement, set up payment schedules, and provide the borrower with a detailed explanation of the terms.
4.4 Continuous Monitoring and Adjustments
Even after the credit has been approved, automated systems continue to monitor a borrower’s repayment behavior. AI models can track payment histories, flag late payments, and predict potential defaults, enabling lenders to take proactive measures, such as offering payment extensions or restructuring loans, to mitigate risk.
5. Challenges of Automation in Credit Approvals
How Automation Is Speeding Up, there are some challenges and limitations to consider:
5.1 Data Privacy and Security
Automated systems often rely on large amounts of personal and financial data. Ensuring the privacy and security of this information is paramount. Financial institutions must implement strong encryption protocols, secure data storage, and compliance with data protection regulations (such as GDPR or CCPA) to protect consumer information.
5.2 System Integration and Complexity
Integrating new automated systems with legacy infrastructure can be challenging and costly for financial institutions. Existing systems may not be compatible with new automation tools, requiring significant investment in technology and training.
5.3 Ethical Concerns and Bias
AI and machine learning models are only as good as the data they are trained on. If these systems are trained on biased or incomplete data, they may inadvertently discriminate against certain groups of people. Financial institutions must ensure that their automation models are designed to be fair, transparent, and accountable.